Imagine knowing which customers might leave your service before they do, or understanding exactly when to reach out to a user who’s struggling. This isn’t fortune-telling – it’s the power of predictive analytics in SaaS platforms.
In this article, we’ll explore how SaaS companies are using predictive analytics to make smarter decisions and provide better services to their customers.
What is Predictive Analytics in SaaS?
Before diving into specific use cases, let’s understand what predictive analytics means in the SaaS world.
Think of predictive analytics as a smart assistant that looks at patterns in your data and helps you make educated guesses about what might happen next. Just like how weather forecasters use past weather patterns to predict tomorrow’s weather, SaaS platforms use past user behavior and other data points to predict future outcomes.
For example, when Netflix suggests shows you might like, it’s using predictive analytics to analyze what you’ve watched before and what similar users enjoy. This same principle applies across many different aspects of SaaS businesses.
Customer Churn Prediction and Prevention
One of the most valuable applications of predictive analytics is identifying customers who might stop using your service. Think of it as an early warning system for your business.
How it works in practice:
- The system continuously monitors user behavior patterns, such as login frequency, feature usage, and engagement levels
- It compares these patterns with those of customers who have left in the past
- When similar patterns emerge, the system alerts the customer success team
- Teams can then take proactive steps to re-engage these customers before they leave
Usage Pattern Analysis and Resource Optimization
Predictive analytics helps SaaS companies understand and prepare for how customers will use their platform. This is similar to how grocery stores stock up on ice cream during summer months because they can predict increased demand.
Real-world applications include:
- Predicting peak usage times to allocate server resources efficiently
- Forecasting storage needs for different customer segments
- Anticipating support team staffing requirements based on usage patterns
- Optimizing feature development based on predicted user needs
Personalized User Experience and Feature Recommendations
Just as a good salesperson remembers your preferences and suggests products you might like, predictive analytics helps SaaS platforms create personalized experiences for each user.
This manifests in several ways:
- Custom dashboard layouts based on predicted user preferences
- Feature suggestions based on user role and behavior
- Personalized onboarding paths for new users
- Tailored content and help resources
Revenue Prediction and Pricing Optimization
Predictive analytics helps SaaS companies understand their financial future and optimize their pricing strategies. Think of it as having a financial advisor who can see patterns in customer behavior and spending.
Applications include:
- Forecasting monthly recurring revenue (MRR)
- Identifying upsell opportunities
- Predicting customer lifetime value
- Optimizing pricing tiers based on usage patterns
Customer Support Optimization
Practical applications include:
- Predicting which customers are likely to need help
- Anticipating peak support times
- Suggesting solutions based on past similar issues
- Routing tickets to the most qualified support agents
Product Development and Feature Planning
Predictive analytics helps product teams make data-driven decisions about what to build next. It’s similar to how city planners use traffic patterns to decide where to build new roads.
This includes:
- Identifying which features are likely to have the highest impact
- Predicting adoption rates for new features
- Understanding which parts of the product might need improvement
- Forecasting the resources needed for development
Implementation Steps for SaaS Companies
If you’re considering implementing predictive analytics in your SaaS platform, here’s a practical approach to getting started:
Start with Data Collection
First, ensure you’re collecting the right data:
- User behavior metrics
- Customer feedback and support interactions
- Usage patterns and feature adoption rates
- Billing and subscription data
Choose Your Focus Area
Begin with one specific use case that could provide immediate value:
- If customer churn is a concern, start with churn prediction
- If resource optimization is crucial, focus on usage pattern analysis
- If personalization is a priority, begin with user experience analytics
Build Your Analytics Infrastructure
Develop the necessary systems:
- Data storage and processing capabilities
- Analysis tools and algorithms
- Reporting and visualization systems
- Alert and notification mechanisms
Test and Refine
Implement in phases:
- Start with a small user segment
- Measure the accuracy of predictions
- Gather feedback from teams using the insights
- Adjust and improve based on results
Best Practices and Considerations
To make the most of predictive analytics in your SaaS platform:
Privacy and Security:
- Ensure compliance with data protection regulations
- Be transparent with users about data collection
- Implement strong data security measures
- Allow users to control their data sharing preferences
Data Quality:
- Regularly validate data accuracy
- Clean and normalize data consistently
- Document data collection methods
- Maintain data governance standards
Team Collaboration:
- Share insights across departments
- Train teams on using predictive analytics tools
- Create clear processes for acting on predictions
- Establish feedback loops for continuous improvement
Conclusion
Predictive analytics is transforming how SaaS platforms operate, from improving customer experiences to optimizing business operations. By starting small, focusing on specific use cases, and following best practices, SaaS companies can harness the power of predictive analytics to make better decisions and provide more value to their customers.
Remember, the goal isn’t to predict the future perfectly, but to make more informed decisions based on data-driven insights. Start with one area where predictive analytics could provide immediate value to your business, and expand from there as you see results.
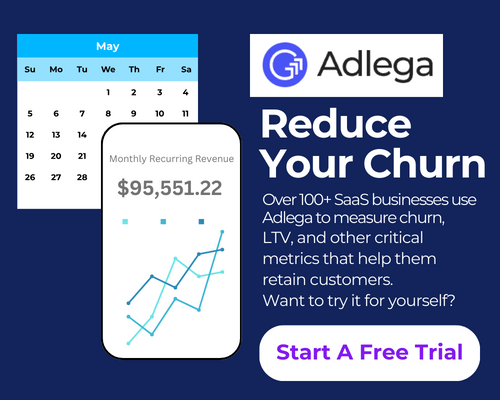
Leave a Reply